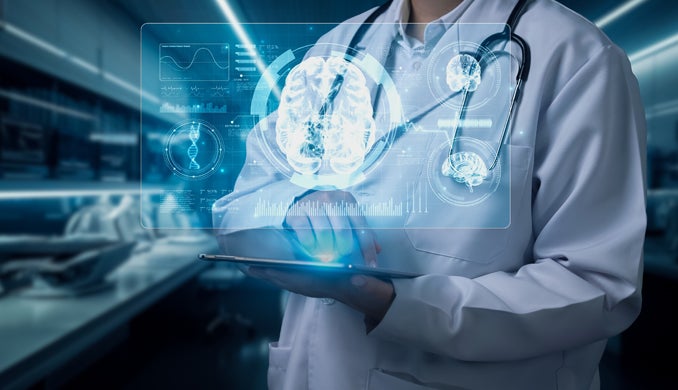
Neurodevelopmental disorders (NDDs), including attention deficit hyperactivity disorder (ADHD) and autism spectrum disorder (ASD), represent a complex and growing global challenge. These disorders often co-occur with mental health conditions in childhood, exacerbating difficulties for affected individuals, their families, and wider society. In Qatar, a recent study reported an ADHD prevalence of 808 to 1,550 per 100,000 people, with a higher rate in males. There is no standardized physical or biological test for ADHD or ASD, and diagnosis of some affected individuals, such as adults with ADHD, can take up to 7 years.
Challenges in Current Diagnostic Methods and the Need for Innovative Solutions
The current diagnostic methods for ADHD and ASD have two disadvantages. Both rely heavily on the clinician's training and experience while lacking objective measures, leading to inconsistencies in outcomes due to the absence of standardized measurements. A recent study reported a very low inter-rater reliability among clinicians diagnosing ADHD. Many tests do not provide clear biological markers, leading to misdiagnosis especially in adolescents whose self-reports may be unreliable.
Traditional diagnostic processes are also time-consuming thereby delaying treatment and risking patient health. AI can address these issues by rapidly analyzing vast datasets to provide quicker preliminary diagnoses and identifying critical cases. Additionally, AI enhances objectivity by integrating diverse data sources, revealing patterns and biomarkers that improve accuracy.
Researchers in the Neurological Disorders Institute at Hamad Bin Khalifa University’s (HBKU) Qatar Biomedical Research Institute (QBRI) are committed to advancing biomarker discovery in NDDs. The integration of AI into diagnostic processes represents a key innovation that can transform the diagnostic process of ADHD and ASD, making diagnosis faster and more accurate, and helping to tailor individual treatment plans. This Insight provides an overview of advances in AI that support the diagnosis of ADHD and ASD, while highlighting new technologies that leverage Omics-based studies for better detection of biomarkers associated with these disorders.
AI Technologies in Behavioral Assessment
AI has introduced sophisticated tools such as machine learning (ML) and natural language processing (NLP), which hold great potential for enhancing the diagnostic process for NDDs. ML algorithms can be trained on large datasets of behavioral observations to identify patterns indicative of different NDDs like ADHD or ASD. These algorithms can analyze and interpret different inputs including video recordings to detect atypical behaviors associated with different disorders.
A recent study by Ara et al. (2024) evaluated ML algorithms for the early diagnosis of ASD. It used video data and managed to achieve an accuracy of 92% in detecting autistic behaviors, such as reduced eye contact and repetitive movements. Similarly, Wall et al. (2018) demonstrated that ML could identify ASD behaviors from home videos with over 94% accuracy. This method not only accelerates the diagnostic process but also improves accessibility, especially for underserved populations. ML can also help in predicting different disorders by detecting patterns in functional MRI scans.
NLP can be employed to analyze speech patterns and language use in individuals with NDDs. Research has shown that children with ASD often exhibit distinct speech patterns, such as delayed language development, a behavior known as echolalia (repeating words or phrases they hear), and atypical prosody (unusual rhythm or pitch in speech). Quantitative analysis of texts produced by diagnosed individuals, which can often be biased or inconsistent, helps improve early identification and diagnosis. NLP tools can analyze speech patterns and language use to identify communication deficits often seen in ASD. By quantifying and analyzing these features, NLP can aid in diagnosis.
A study by Themistocleous et al. (2024) used NLP to analyze speech from children with ASD and achieved 96% accuracy in distinguishing them from their neurotypical peers. Similarly, NLP has been used to enhance ADHD diagnosis through linguistic analysis. Stylometric techniques have been applied to identify "linguistic fingerprints" that differentiate individuals with ADHD from those without the disorder. The primary advantage of AI in behavioral assessment is its ability to process large volumes of data efficiently, enabling early detection of atypical behaviors and reducing the subjective biases inherent in human observations.
Integrating 'Omic' Studies with AI
Recent advancements in 'Omic' technologies, such as genomics, transcriptomics, proteomics, and metabolomics, have deepened our understanding of the biological markers associated with ADHD and ASD. AI, particularly machine and deep learning, has proven invaluable in analyzing multi-omics data to uncover complex biological patterns (Figure 1).
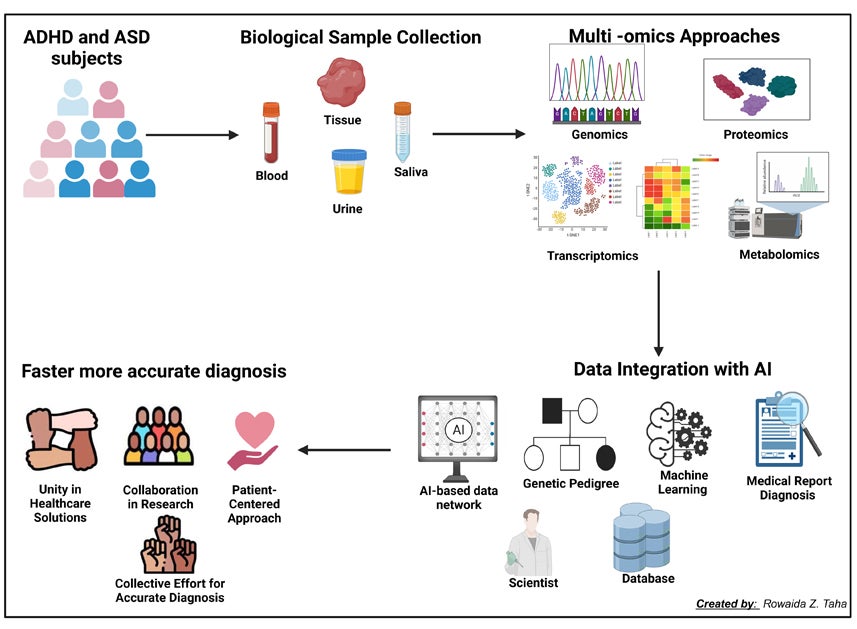
Genome-wide association studies (GWAS) have identified several genetic variants associated with ADHD and ASD. For example, genes such as DAT1, DRD4, and SLC6A3 are involved in dopamine transport, which is relevant to ADHD, while CHD8 and NRXN1 are implicated in synaptic function in ASD. AI can assist in processing these vast genomic datasets to identify genetic risk factors and their interaction with environmental influences.
Transcriptomics studies provide insights into gene expression patterns, which can shed light on the molecular mechanisms underlying ADHD and ASD. Nahas et al. (2022) identified over 3,000 differentially expressed genes (DEGs) in individuals with ASD, highlighting their potential as biomarkers. AI-driven analyses of transcriptomic data can further refine these findings, revealing the molecular networks involved in neurodevelopmental disorders.
The meta-analysis conducted by Nahas et al. identified 342 DEGs with an adjusted p-value of ≤ 0.001, further refining the search for potential biomarkers. This study also utilized text mining techniques to identify genes with previously established links to neurodevelopmental genes, such as MAPK8IP3 and SSH1. These findings highlight the potential of transcriptomic data to reveal the intricate molecular networks involved in ADHD and ASD.
Proteomics and metabolomics offer complementary insights into the biochemical pathways involved in ADHD and ASD. Proteomic studies have identified changes in synaptic proteins and neurotransmitter systems, while metabolomics studies have highlighted metabolic signatures linked to neurotransmitter dysregulation and oxidative stress. AI can integrate these datasets, identifying novel biomarkers for early diagnosis and personalized treatment.
AI’s role in analyzing multi-omics data is transformative, enabling researchers to uncover previously hidden relationships between genetic, transcriptomic, and proteomic data. For example, a study by Zhang et al. (2023) demonstrated how ML could integrate genetic, neurobiological, and neuroimaging data to improve diagnostic accuracy for ADHD. AI’s ability to process complex data in real time facilitates the identification of biomarkers that could enhance early diagnosis, monitor disease progression, and evaluate treatment efficacy.
The integration of AI with modern 'Omic' studies has the potential to revolutionize the way we diagnose and treat NDDs like ADHD and ASD. By reducing the subjectivity of traditional methods and enhancing early detection through data-driven tools, AI paves the way for more accurate, personalized, and timely treatments.
Contributed by:
Rowaida Z. Taha (Senior Research Associate, QBRI) & Dr. Salam Salloum-Asfar (Postdoctoral Researcher, QBRI).
Arabic text validation: Rowaida Z. Taha (Senior Research Associate, QBRI).